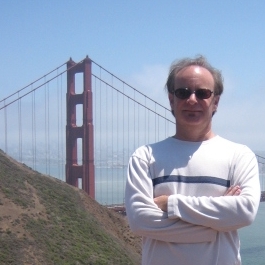
The End of Software
Will Programming Become Obsolete?
by Kevin Morris, Electronic Engineering Journal
Tell a crowd of nerds that software is coming to an end, and you’ll get laughed out of the bar. The very notion that the amount of software and software development in the world will do anything besides continue on an exponential growth curve is unthinkable in most circles. Examine any industry data and you’ll see the same thing – software content is up and to the right. For decades, the trend in system design has been toward increasing the proportion of functionality implemented in software versus hardware, and the makeup of engineering teams has followed suit. It is not uncommon these days to see a 10:1 ratio of software versus hardware engineers on an electronic system development project. And that doesn’t count the scores of applications developed these days that are software alone.
Yep, it’s all coming to an end.
But, software is one of those five immutable elements, isn’t it – fire, water, earth, air, and code? Practically nothing exists in our technological world today without software. Rip out software and you have taken away the very essence of technology – its intelligence – its functionality – its soul.
Is software really all that important?
Let’s back up a bit. Every application, every system is designed to solve a problem. The solution to that problem can generally be broken down into two parts: the algorithm and the data. It is important to understand that the actual answer always lies within the data. The job of the algorithm is simply to find that answer amongst the data as efficiently as possible. Most systems today further break the algorithm portion of that down into two parts: hardware and software. Those elements, of course, form the basis of just about every computing system we design – which comprises most of modern technology.
We all know that, if the algorithm is simple enough, you can sometimes skip the software part. Many algorithms can be implemented in hardware that processes the data and finds the solution directly, with no software required. The original hand calculators, the early arcade video games, and many other “intelligent” devices have used this approach. If you’re not doing anything more complex than multiplication or maybe the occasional quadratic formula, bring on the TTL gates!
The problem with implementing algorithms directly in hardware, though, is that the complexity of the hardware scales with the complexity of the algorithm. Every computation or branch takes more physical logic gates. Back in the earliest days of Moore’s Law, that meant we had very, very tight restrictions on what could be done in optimized hardware. It didn’t take much complexity to strain the limits of what we were willing and able to solder onto a circuit board with discrete logic gates. “Pong” was pushing it. Anything more complicated than that and we leapt blissfully from the reckless optimism of Moore into the warm and comfortable arms of Turing and von Neumann.
The conventional von Neumann processor architecture uses “stored programs” (yep, there it is, software) to allow us to handle arbitrarily complex algorithms without increasing the complexity of our hardware. We can design one standard piece of hardware – the processor, and using that we can execute any number of arbitrarily complex algorithms. Hooray!
But every good thing comes at a price, doesn’t it? The price of programmability is probably somewhere between three and four orders of magnitude. Yep. Your algorithm might run somewhere between 100 and 10,000 times as fast if it were implemented in optimized hardware compared with software on a conventional processor. All of that business with program counters and fetching instructions is really a lot of overhead on top of the real work. And, because of the sequential nature of software, pure von Neumann machines trudge along doing things one at a time that could easily be done simultaneously.
The performance cost of software programmability is huge, but the benefits are, as well. Both hardware cost and engineering productivity are orders of magnitude better. An algorithm you could implement in a few hours in software might require months of development time to create in optimized hardware – if you could do it at all. This tradeoff is so attractive, in fact, that the world has spent over half a century optimizing it. And, during that half century, Moore’s Law has caused the underlying hardware implementation technology to explode exponentially.
The surface of software development has remained remarkably calm considering the turbulence created by Moore’s Law below. The number of components we can cram onto integrated circuits has increased by something like eight orders of magnitude since 1965, and yet we still write and debug code one line at a time. Given tens of millions of times the transistors, processors have remained surprisingly steady as well. Evolution has taken microprocessors from four to sixty-four bits, from one core to many, and has added numerous performance-enhancing features such as caches, pipelines, hardware arithmetic, predictive execution, and so forth, but the basic von Neumann architecture remains largely unchanged.
It’s important to note that all of the enhancements to processor architecture have done – all they ever can do – is to mitigate some of the penalty of programmability. We can make a processor marginally closer to the performance of optimized hardware, but we can never get there – or even close.
Of course, those eight orders of magnitude Moore’s Law has given us in transistor count also mean that the complexity of the algorithms we could implement directly in optimized hardware has similarly increased. If you’re willing and able to make a custom optimized chip to run your specific algorithm, it will always give 100x or more the performance of a software version running on a processor using similarly less energy. The problem there, of course, is the enormous cost and development effort required. Your algorithm has to be pretty important to justify the ~24-month development cycle and most likely eight-figure investment to develop an ASIC.
During that same time, however, we have seen the advent of programmable hardware, in the form of devices such as FPGAs. Now, we can implement our algorithm in something like optimized hardware using a general purpose device (an FPGA) with a penalty of about one order of magnitude compared with actual optimized hardware. This has raised the bar somewhat on the complexity of algorithms (or portions thereof) that can be done without software. In practical terms, however, the complexity of the computing problems we are trying to solve has far outpaced our ability to do them in any kind of custom hardware – including FPGAs. Instead, FPGAs and ASICs are relegated to the role of “accelerators” for key computationally intensive (but less complex) portions of algorithms that are still primarily implemented in software.
Nevertheless, we have a steadily rising level of algorithmic complexity where solutions can be implemented without software. Above that level – start coding. But there is also a level of complexity where software starts to fail as well. Humans can create algorithms only for problems they know how to solve. Yes, we can break complex problems down hierarchically into smaller, solvable units and divide those portions up among teams or individuals with various forms of expertise, but when we reach a problem we do not know how to solve, we cannot write an algorithm to do it.
As a brilliant engineer once told me, “There is no number of 140 IQ people that will replace a 160 IQ person.” OK, this person DID have a 160 IQ (so maybe there was bias in play) but the concept is solid. We cannot write a program to solve a problem we are not smart enough to solve ourselves.
Until AI.
With deep learning, for example, we are basically bypassing the software stage and letting the data itself create the algorithm. We give our system a pile of data and tell it the kind of answer we’re looking for, and the system itself divines the method. And, in most cases, we don’t know how it’s doing it. AI may be able to look at images of a thousand faces and tell us accurately which ones are lying, but we don’t know if it’s going by the angle of the eyebrows, the amount of perspiration, the curl of the lip, or the flaring of the nostrils. Most likely, it is a subtle and complex combination of those.
We now have the role of software bounded – both on the low- and high-complexity sides. If the problem is too simple, it may be subsumed by much more efficient dedicated hardware. If it’s too complex, it may be taken over by AI at a much lower development cost. And the trendlines for each of those is moving toward the center – slowly reducing the role of classical software.
As we build more and more dedicated hardware to accelerate high compute load algorithms, and as we evolve the architectures for AI to expand the scope of problems it can solve, we could well slowly reduce the role of traditional software to nothing. We’d have a world without code.
I wouldn’t go back to bartending school just yet, though. While the von Neumann architecture is under assault from all sides, it has a heck of a moat built for itself. We are likely to see the rapid acceleration of software IP and the continued evolution of programming methods toward higher levels of abstraction and increased productivity for software engineers. Data science will merge with computer science in yet-unforeseen ways, and there will always be demanding problems for bright minds to solve. It will be interesting to watch.
This article first appeared at EE Journal on April 18, 2018.
No Comments