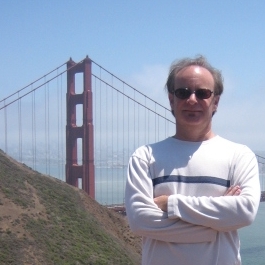
Machine Learning vs. AI, Important Differences Between
Unfortunately, some tech organizations are deceiving customers by proclaiming using AI on their technologies while not being clear about their products’ limits
By Roberto Iriondo
August 23, 2019
Recently, a report was released regarding the misuse from companies claiming to use artificial intelligence on their products and services. According to the Verge, 40% of European startups that claimed to use AI don’t actually use the technology. Last year, TechTalks, also stumbled upon such misuse by companies claiming to use machine learning and advanced artificial intelligence to gather and examine thousands of users’ data to enhance user experience in their products and services.
Unfortunately, there’s still a lot of confusion within the public and the media regarding what truly is artificial intelligence, and what truly is machine learning. Often the terms are being used as synonyms, in other cases, these are being used as discrete, parallel advancements, while others are taking advantage of the trend to create hype and excitement, as to increase sales and revenue.
Below we will go through some main differences between AI and machine learning.
What is machine learning?
Quoting Interim Dean at the School of Computer Science at CMU, Professor and Former Chair of the Machine Learning Department at Carnegie Mellon University, Tom M. Mitchell:
A scientific field is best defined by the central question it studies. The field of Machine Learning seeks to answer the question:
“How can we build computer systems that automatically improve with experience, and what
are the fundamental laws that govern all learning processes?”
Machine learning (ML) is a branch of artificial intelligence, and as defined by Computer Scientist and machine learning pioneer Tom M. Mitchell: “Machine learning is the study of computer algorithms that allow computer programs to automatically improve through experience.” — ML it’s one of the ways we expect to achieve AI. Machine learning relies on working with small to large data-sets by examining and comparing the data to find common patterns and explore nuances.
For instance, if you provide a machine learning model with a lot of songs that you enjoy, along their corresponding audio statistics (dance-ability, instrumentality, tempo or genre), it will be able to automate (depending of the supervised machine learning model used) and generate a recommender system as to suggest you with music in the future that (with a high percentage of probability rate) you’ll enjoy, similarly as to what Netflix, Spotify, and other companies do.
In a simple example, if you load a machine learning program with a considerable large data-set of x-ray pictures along with their description (symptoms, items to consider, etc.), it will have the capacity to assist (or perhaps automatize) the data analysis of x-ray pictures later on. The machine learning model will look at each one of the pictures in the diverse data-set, and find common patterns found in pictures that have been labeled with comparable indications. Furthermore, (assuming that we use a good ML algorithm for images) when you load the model with new pictures it will compare its parameters with the examples it has gathered before in order to disclose to you how likely the pictures contain any of the indications it has analyzed previously.
Supervised Learning (Classification/Regression) | Unsupervised Learning (Clustering) | Credits: Western Digital
The type of machine learning from our previous example is called “supervised learning,” where supervised learning algorithms try to model relationship and dependencies between the target prediction output and the input features, such that we can predict the output values for new data based on those relationships, which it has learned from previous data-sets fed.
Unsupervised learning, another type of machine learning are the family of machine learning algorithms, which are mainly used in pattern detection and descriptive modeling. These algorithms do not have output categories or labels on the data (the model is trained with unlabeled data).
No Comments